Reports
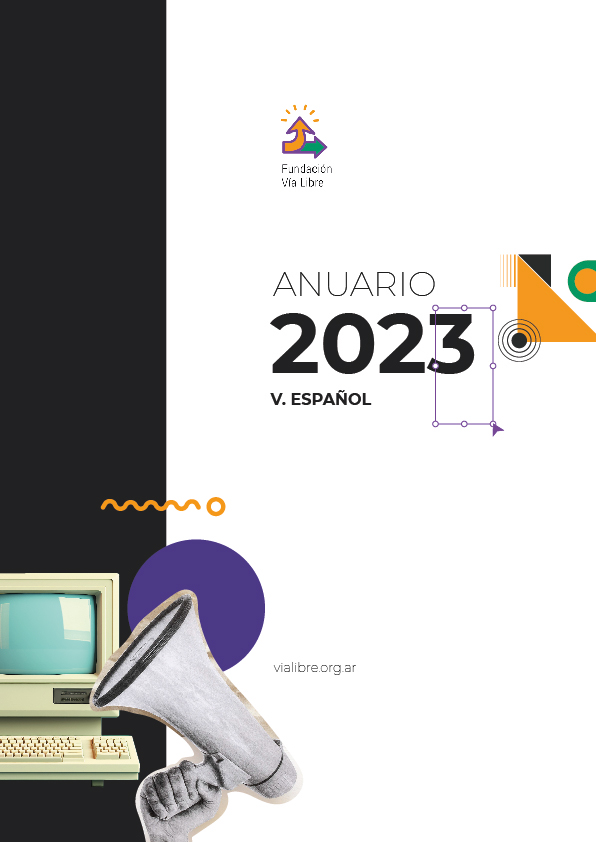
Yearbook 2023
We share the summary of the activities of Foundation Vía Libre
during the year 2023.
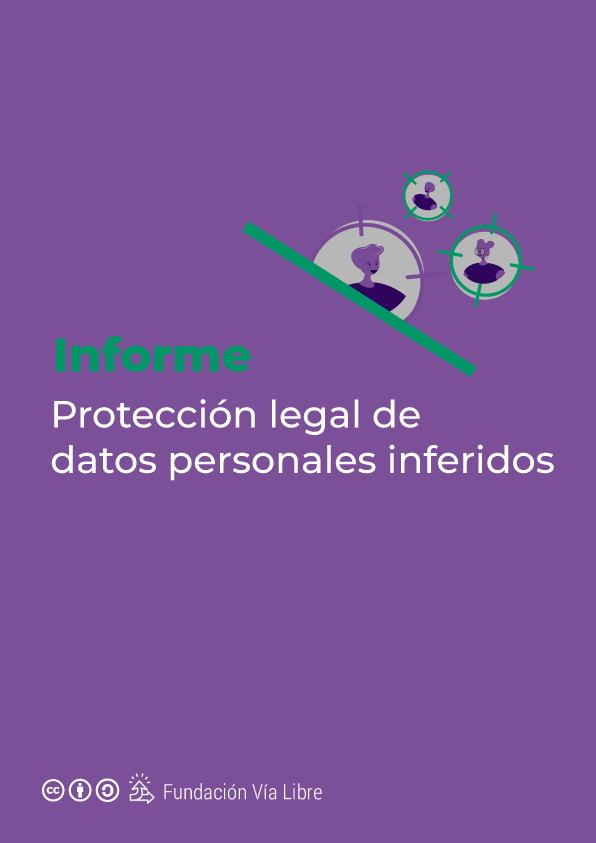
Legal protection of inferred personal data
In this report we address the legal protection of inferred data from its production to its use and impact, aiming to recognise its existence and account for its impact on everyday life. Through some examples, we see how they are the basis for decisions that can affect everything from privacy to autonomy and potentially the physical integrity of individuals. In view of this, we briefly review the regulatory situation in other countries in order to finally consider a possible regulation in Argentina that would guarantee rights.
We seek through this document to reflect on the importance of understanding that inferred data are personal data, and as such should be regulated from a human rights perspective.
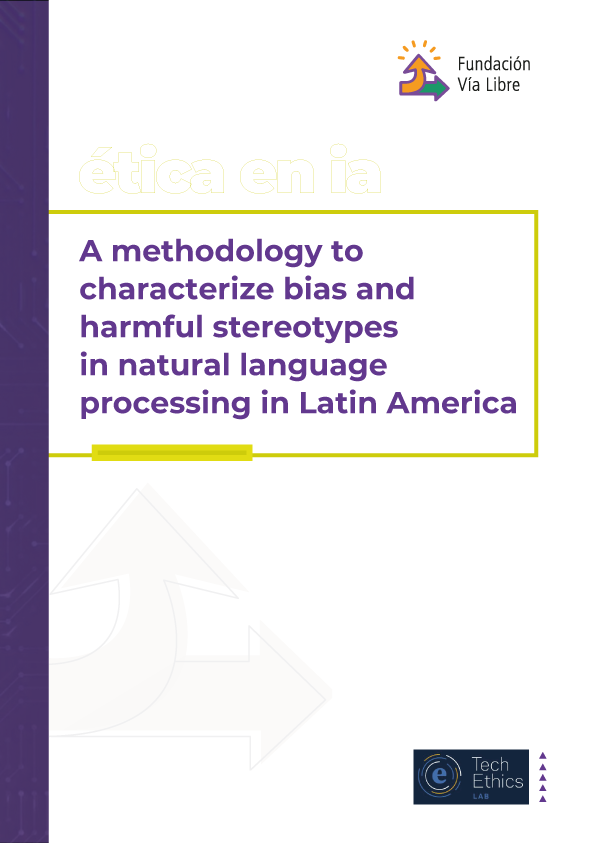
A methodology to characterize bias and harmful stereotypes in natural language processing in Latin America
In this paper we present a methodology that spells out how social scientists, domain experts, and machine learning experts can collaboratively explore biases and harmful stereotypes in word embeddings and large language models. Our methodology uses the software we implemented, available at https://huggingface.co/spaces/vialibre/edia
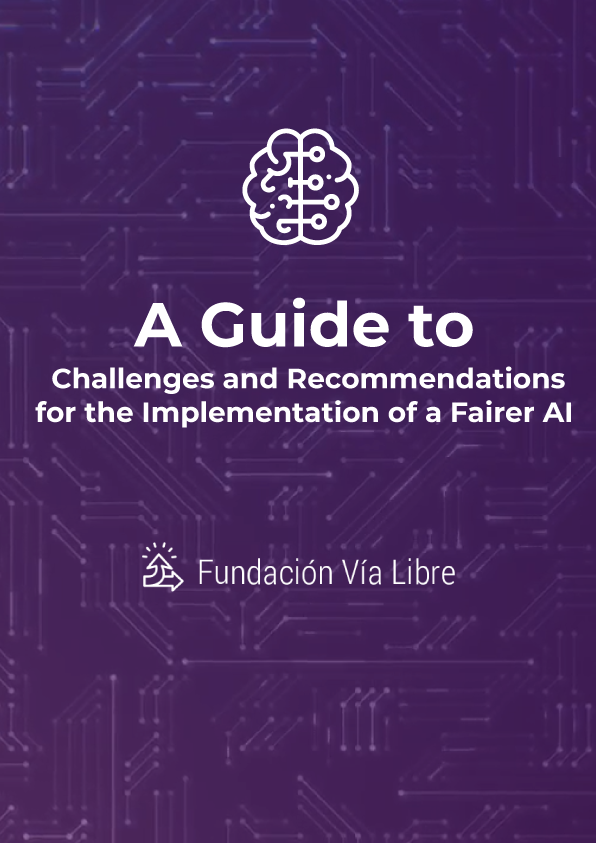
A Guide to Challenges and Recommendations for the Implementation of a Fairer AI
In this document, we elaborated a list of technical recommendations for the development of Artificial Intelligence (AI) projects, specifically Machine Learning based systems. These recommendations are the result of structured interviews with people who work in practical applications of data-based systems in various roles and organizations within the Argentine technological ecosystem, and have been elaborated through the lens of our AI ethics team, composed of activists, social scientists and computer science researchers.
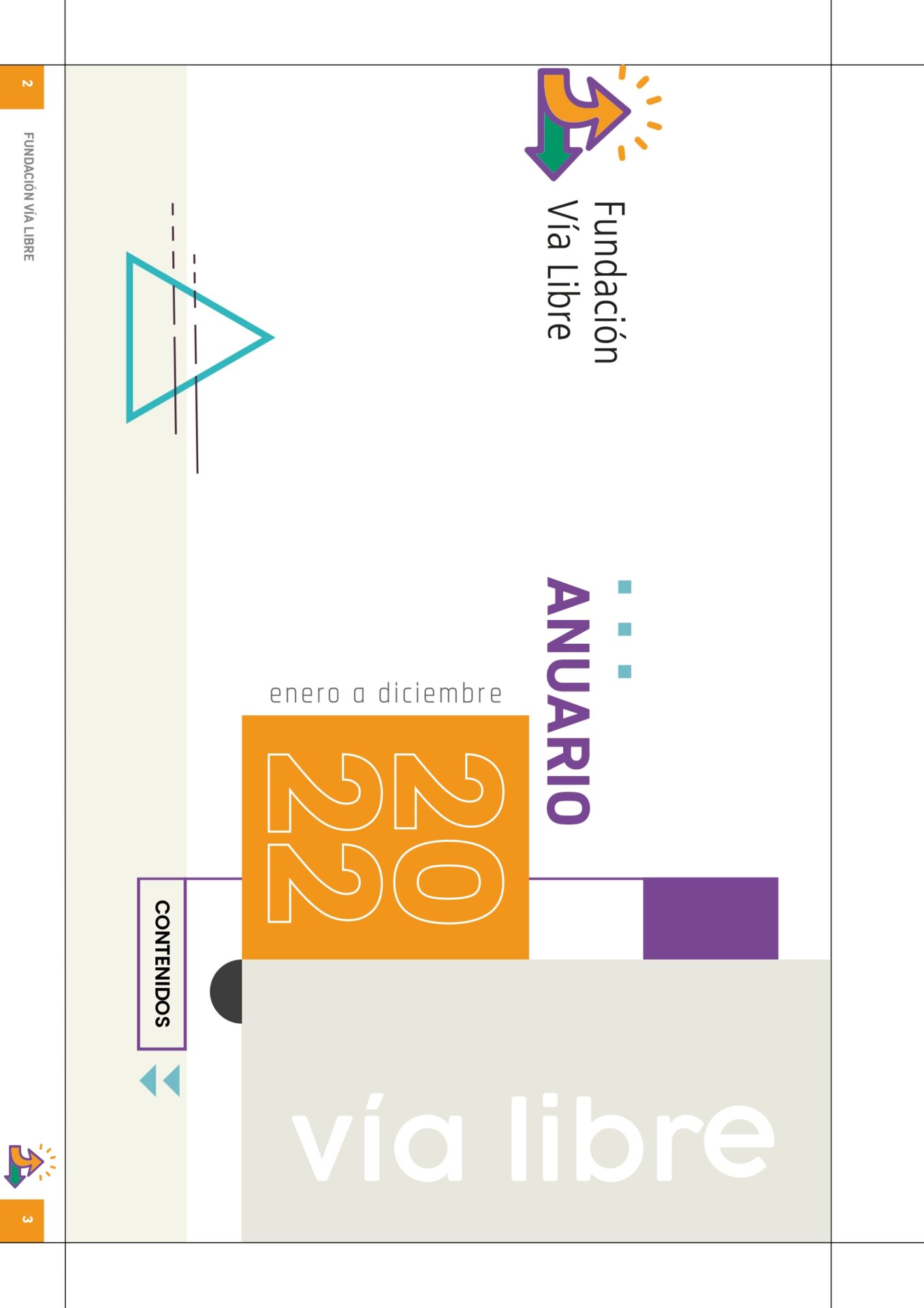
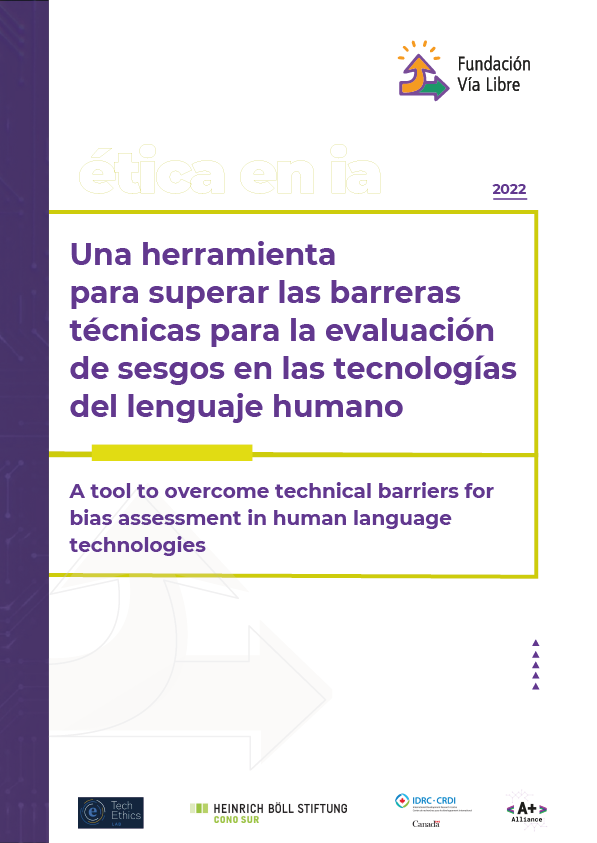
A tool to overcome technical barriers for bias assessment in human language technologies
Automatic processing of language is becoming pervasive in our lives, often taking central roles in our decision making, like choosing the wording for our messages and mails, translating our readings, or even having full conversations with us. Word embeddings are a key component of modern natural language processing systems. They provide a representation of words that has boosted the performance of many applications, working as a semblance of meaning.
Word embeddings seem to capture a semblance of the meaning of words from raw text, but, at the same time, they also distill stereotypes and societal biases which are subsequently relayed to the final applications. Such biases can be discriminatory. It is very important to detect and mitigate those biases, to prevent discriminatory behaviors of automated processes, which can be much more harmful than in the case of humans because their of their scale. There are currently many tools and techniques to detect and mitigate biases in word embeddings, but they present many barriers for the engagement of people without technical skills. As it happens, most of the experts in bias, either social scientists or people with deep knowledge of the context where bias is harmful, do not have such skills, and they cannot engage in the processes of bias detection because of the technical barriers.
We have studied the barriers in existing tools and have explored their possibilities and limitations with different kinds of users. With this exploration, we propose to develop a tool that is specially aimed to lower the technical barriers and provide the exploration power to address the requirements of experts, scientists and people in general who are willing to audit these technologies.
A tool to overcome technical barriers for bias assessment in human language technologies